This paper presents a method for object recognition once parts have been detected. The recognition task is formulated as a graph problem searching for the characteristic geographical arrangements of (possibly missing) parts. The objective function is Bayesian maximum a posteriori estimation, integrating the image likelihood as a posteriori probability of the part detectors. The variability in the arrangement of object parts is captured by a Gaussian distribution after translation normalization. By employing two special properties of a Gaussian distribution, we are able to deal with missing parts situation where the chosen origin is not detected. We use an A* algorithm to find the optimal solution for the graph search problem. Experiments are performed on both synthetic and real data to demonstrate good results and fast performance of the recognition.
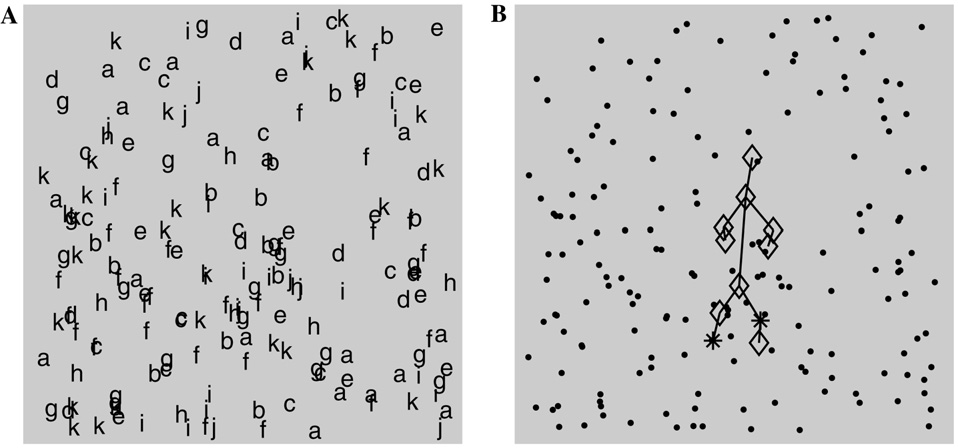
@Article{PhamCVIU2005,
author = "Pham, T. V. and Smeulders, A. W. M.",
title = "Object Recognition with Uncertain Geometry and Uncertain Part Detection",
journal = "Computer Vision and Image Understanding",
number = "2",
volume = "99",
pages = "241--258",
year = "2005",
url = "https://ivi.fnwi.uva.nl/isis/publications/2005/PhamCVIU2005",
pdf = "https://ivi.fnwi.uva.nl/isis/publications/2005/PhamCVIU2005/PhamCVIU2005.pdf",
has_image = 1
}