The paper is concerned with two-class active learning. While the common approach for collecting data in active learning is to select samples close to the classification boundary, better performance can be achieved by taking into account the prior data distribution. The main contribution of the paper is a formal framework that incorporates clustering into active learning. The algorithm first constructs a classifier on the set of the cluster representatives, and then propagates the classification decision to the other samples via a local noise model. The proposed model allows to select the most representative samples as well as to avoid repeatedly labeling samples in the same cluster. During the active learning process, the clustering is adjusted using the coarse-to-fine strategy in order to balance between the advantage of large clusters and the accuracy of the data representation. The results of experiments in image databases show a better performance of our algorithm compared to the current methods.
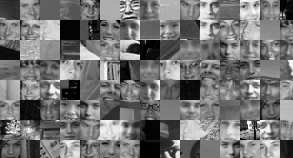
@InProceedings{NguyenICML2004,
author = "Nguyen, H. T. and Smeulders, A. W. M.",
title = "Active Learning Using Pre-Clustering",
booktitle = "International Conference on Machine Learning",
pages = "623--630",
year = "2004",
url = "https://ivi.fnwi.uva.nl/isis/publications/2004/NguyenICML2004",
pdf = "https://ivi.fnwi.uva.nl/isis/publications/2004/NguyenICML2004/NguyenICML2004.pdf",
has_image = 1
}