Learning classifiers for many visual concepts is important for image categorization and retrieval. As a classifier tends to misclassify negative examples which are visually similar to positive ones, inclusion of such misclassified and thus relevant negatives should be stressed during learning. User-tagged images are abundant online, but
which images are the relevant negatives remains unclear. Sampling negatives at random is the de facto standard in the literature. In this paper we go beyond random sampling by proposing Negative Bootstrap. Given a visual concept and a few positive examples, the new algorithm iteratively finds relevant negatives. Per iteration we learn from a small proportion of many user-tagged images, yielding an ensemble of meta classifiers. For efficient classification, we introduce Model Compression such that the classification time is independent of the ensemble size. Compared to the state of the art, we obtain relative gains of 14% and 18% on two present-day benchmarks in terms of mean average precision. For concept search in one million images, model compression reduces the search time from over 20 hours to approximately 6 minutes. The effectiveness and efficiency, without the need of manually labeling any negatives, make negative bootstrap appealing for learning better visual concept classifiers.
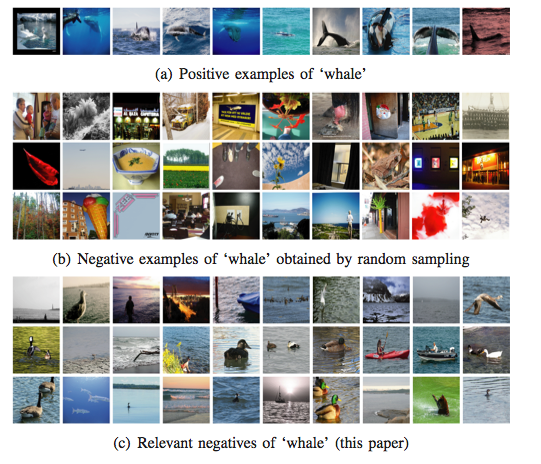
@Article{LiITM2013,
author = "Li, X. and Snoek, C. G. M. and Worring, M. and Koelma, D. C.
and Smeulders, A. W. M.",
title = "Bootstrapping Visual Categorization with Relevant Negatives",
journal = "IEEE Transactions on Multimedia",
number = "4",
volume = "15",
pages = "933--945",
year = "2013",
url = "https://ivi.fnwi.uva.nl/isis/publications/2013/LiITM2013",
pdf = "https://ivi.fnwi.uva.nl/isis/publications/2013/LiITM2013/LiITM2013.pdf",
has_image = 1
}