Social image analysis and retrieval is important for helping people
organize and access the increasing amount of user-tagged multimedia.
Since user tagging is known to be uncontrolled, ambiguous, and
overly personalized, a fundamental problem is how to interpret the
relevance of a user-contributed tag with respect to the visual
content the tag is describing. Intuitively, if different persons
label visually similar images using the same tags, these tags are
likely to reflect objective aspects of the visual content. Starting
from this intuition, we propose in this paper a neighbor voting
algorithm which accurately and efficiently learns tag relevance by
accumulating votes from visual neighbors. Under a set of well
defined and realistic assumptions, we prove that our algorithm is a
good tag relevance measurement for both image ranking and tag
ranking.
Three experiments on 3.5 million Flickr photos demonstrate
the general applicability of our algorithm in both social image
retrieval and image tag suggestion. Our tag relevance learning
algorithm substantially improves upon baselines for all the
experiments. The results suggest that the proposed algorithm is
promising for real-world applications.
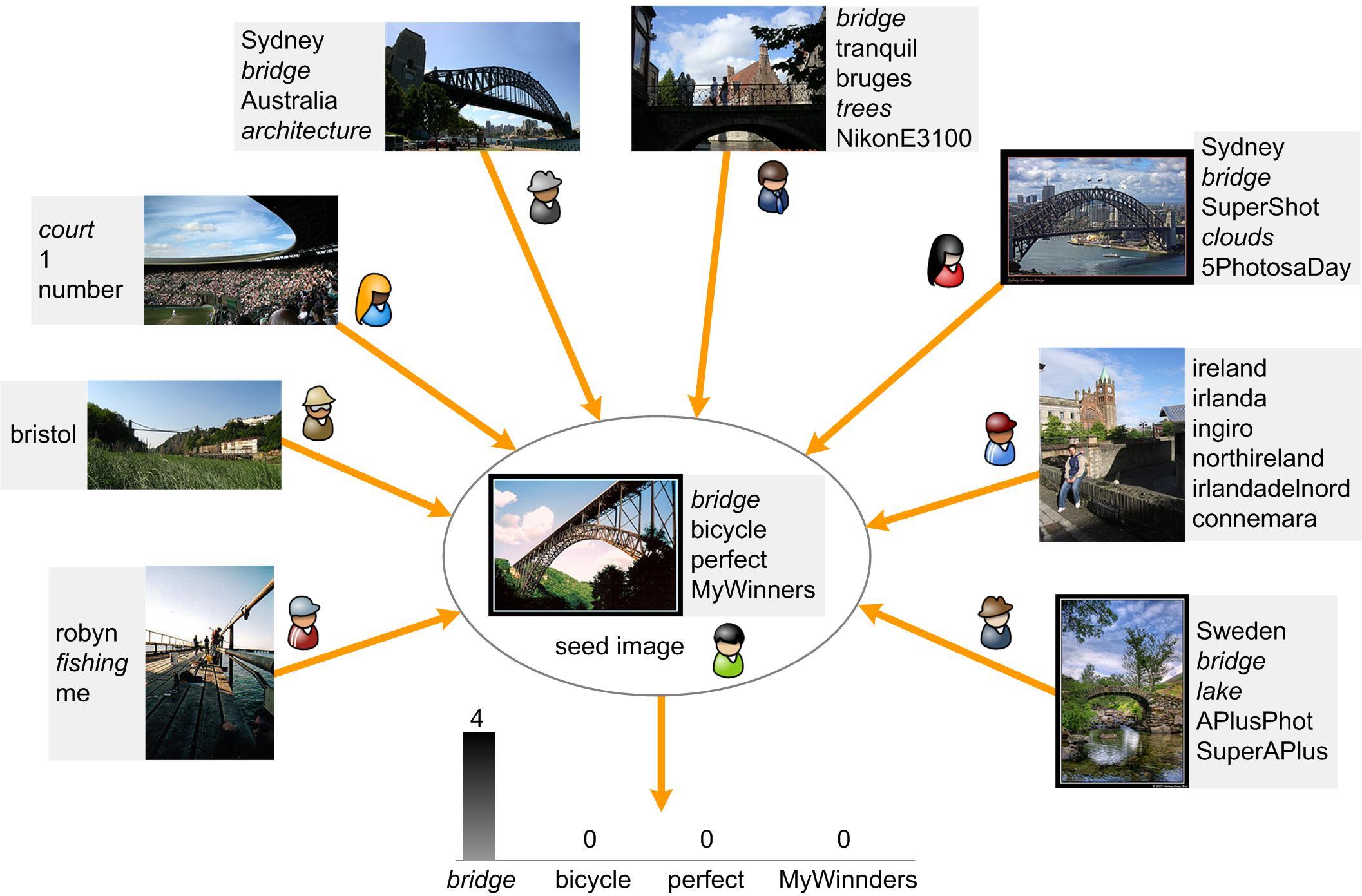
http://staff.science.uva.nl/~xirong/tagrel/
@Article{LiITM2009,
author = "Li, X. and Snoek, C. G. M. and Worring, M.",
title = "Learning Social Tag Relevance by Neighbor Voting",
journal = "IEEE Transactions on Multimedia",
number = "7",
volume = "11",
pages = "1310--1322",
year = "2009",
url = "https://ivi.fnwi.uva.nl/isis/publications/2009/LiITM2009",
pdf = "https://ivi.fnwi.uva.nl/isis/publications/2009/LiITM2009/LiITM2009.pdf",
has_image = 1
}