Vision–based road detection is important in different areas
of computer vision such as autonomous driving, car
collision warning and pedestrian crossing detection. However,
current vision–based road detection methods are usually
based on low–level features and they assume structured
roads, road homogeneity, and uniform lighting conditions.
Therefore, in this paper, contextual 3D information is
used in addition to low–level cues. Low–level photometric
invariant cues are derived from the appearance of
roads. Contextual cues used include horizon lines, vanishing
points, 3D scene layout and 3D road stages. Moreover,
temporal road cues are included. All these cues are sensitive
to different imaging conditions and hence are considered
as weak cues. Therefore, they are combined to improve
the overall performance of the algorithm. To this end, the
low-level, contextual and temporal cues are combined in a
Bayesian framework to classify road sequences.
Large scale experiments on road sequences show that the
road detection method is robust to varying imaging conditions,
road types, and scenarios (tunnels, urban and highway).
Further, using the combined cues outperforms all
other individual cues. Finally, the proposed method provides
highest road detection accuracy when compared to
state–of–the–art methods
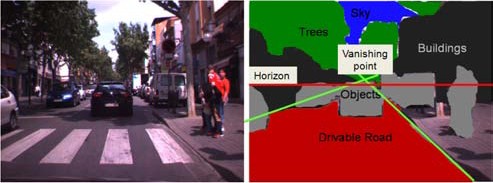
@InProceedings{AlvarezCVPR2010,
author = "Alvarez, J. M. and Gevers, T. and Lopez, A.",
title = "3D Scene Priors for Road Detection",
booktitle = "IEEE Conference on Computer Vision and Pattern Recognition",
pages = "57--64",
year = "2010",
url = "https://ivi.fnwi.uva.nl/isis/publications/2010/AlvarezCVPR2010",
pdf = "https://ivi.fnwi.uva.nl/isis/publications/2010/AlvarezCVPR2010/AlvarezCVPR2010.pdf",
has_image = 1
}