The aim of this paper is fine-grained categorization without human interaction. Different from prior work, which relies on detectors for specific object parts, we propose to localize distinctive details by roughly aligning the objects using just the overall shape. Then, one may proceed to the differential classification by examining the corresponding regions of the alignments. More specifically, the alignments are used to transfer part annotations from training images to unseen images (supervised alignment), or to blindly yet consistently segment the object in a number of regions (unsupervised alignment). We further argue that for the distinction of sub-classes, distribution-based features like color Fisher vectors are better suited for describing localized appearance of fine-grained categories than popular matching oriented intensity features, like HOG. They allow capturing the subtle local differences between subclasses, while at the same time being robust to misalignments between distinctive details. We evaluate the local alignments on the CUB-2011 and on the Stanford Dogs datasets, composed of 200 and 120, visually very hard to distinguish bird and dog species. In our experiments we study and show the benefit of the color Fisher vector parameterization, the influence of the alignment partitioning, and the significance of object segmentation on fine-grained categorization. We, furthermore, show that by using object detectors as voters to generate object confidence saliency maps, we arrive at fully unsupervised, yet highly accurate fine-grained categorization. The proposed local alignments set a new state-of-the-art on both the fine-grained birds and dogs datasets, even without any human intervention. What is more, the local alignments reveal what appearance details are most decisive per fine-grained object category.
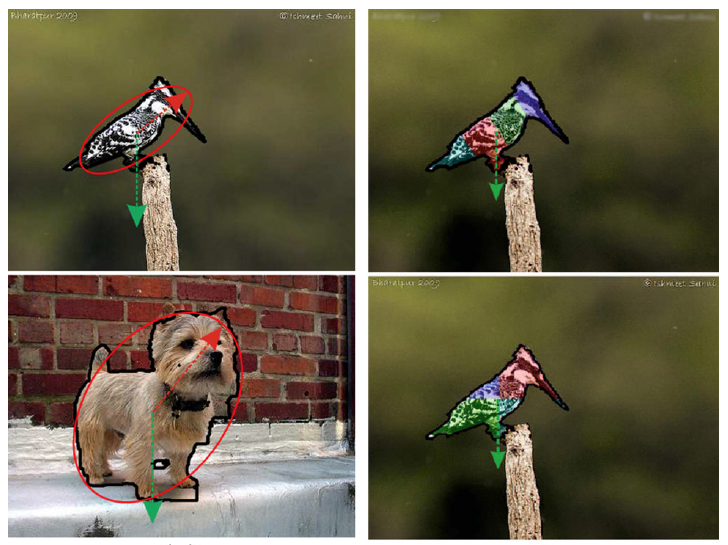
@Article{GavvesIJCV2015,
author = "Gavves, E. and Fernando, B. and Snoek, C. G. M. and Smeulders, A. W. M.
and Tuytelaars, T.",
title = "Local Alignments for Fine-Grained Categorization",
journal = "International Journal of Computer Vision",
year = "2015",
url = "https://ivi.fnwi.uva.nl/isis/publications/2015/GavvesIJCV2015",
pdf = "https://ivi.fnwi.uva.nl/isis/publications/2015/GavvesIJCV2015/GavvesIJCV2015.pdf",
has_image = 1
}