This paper introduces a method for scene categorization by modeling ambiguity in the popular codebook approach. The codebook approach describes an image as a bag of discrete visual codewords, where the frequency distributions of these words are used for image categorization. There are two drawbacks to the traditional codebook model: codeword uncertainty and codeword plausibility. Both of these drawbacks stem from the hard assignment of visual features to a single codeword. We show that allowing a degree of ambiguity in assigning codewords improves categorization performance for three state-of-the-art datasets.
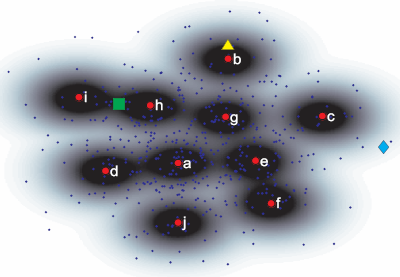
Our results achieved by including this method in the
TRECVID 2008 video retrieval benchmark, and in the
PASCAL-VOC 2008 object categorization benchmark.
@InProceedings{vanGemertECCV2008,
author = "van Gemert, J. C. and Geusebroek, J. M. and Veenman, C. J. and Smeulders, A. W. M.",
title = "Kernel Codebooks for Scene Categorization",
booktitle = "European Conference on Computer Vision",
volume = "3",
pages = "696--709",
year = "2008",
url = "https://ivi.fnwi.uva.nl/isis/publications/2008/vanGemertECCV2008",
pdf = "https://ivi.fnwi.uva.nl/isis/publications/2008/vanGemertECCV2008/vanGemertECCV2008.pdf",
has_image = 1
}