In the face of current large-scale video libraries, the practical applicability of content-based indexing algorithms is constrained by their efficiency. This paper strives for efficient large-scale video indexing by comparing various visual-based concept categorization techniques. In visual categorization, the popular codebook model has shown excellent categorization performance. The codebook model represents continuous visual features by discrete prototypes predefined in a vocabulary. The vocabulary size has a major impact on categorization efficiency, where a more compact vocabulary is more efficient. However, smaller vocabularies typically score lower on classification performance than larger vocabularies. This paper compares four approaches to achieve a compact codebook vocabulary while retaining categorization performance. For these four methods, we investigate the trade-off between codebook compactness and categorization performance. We evaluate the methods on more than 200 h of challenging video data with as many as 101 semantic concepts. The results allow us to create a taxonomy of the four methods based on their efficiency and categorization performance.
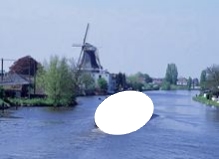
@Article{vanGemertCVIU2010,
author = "van Gemert, J. C. and Snoek, C. G. M. and Veenman, C. J. and Smeulders, A. W. M.
and Geusebroek, J. M.",
title = "Comparing Compact Codebooks for Visual Categorization",
journal = "Computer Vision and Image Understanding",
number = "4",
volume = "114",
pages = "450--462",
year = "2010",
url = "https://ivi.fnwi.uva.nl/isis/publications/2010/vanGemertCVIU2010",
pdf = "https://ivi.fnwi.uva.nl/isis/publications/2010/vanGemertCVIU2010/vanGemertCVIU2010.pdf",
has_image = 1
}