This paper aims for generic instance search from a single example. Where the state-of-the-art relies on global image representation for the search, we proceed by including locality at all steps of the method. As the first novelty, we consider many boxes per database image as candidate targets to search locally in the picture using an efficient point-indexed representation. The same representation allows, as the second novelty, the application of very large vocabularies in the powerful Fisher vector and VLAD to search locally in the feature space. As the third novelty we propose an exponential similarity function to further emphasize locality in the feature space. Locality is advantageous in instance search as it will rest on the matching unique details. We demonstrate a substantial increase in generic instance search performance from one example on three standard datasets with buildings, logos, and scenes from 0.443 to 0.620 in mAP.
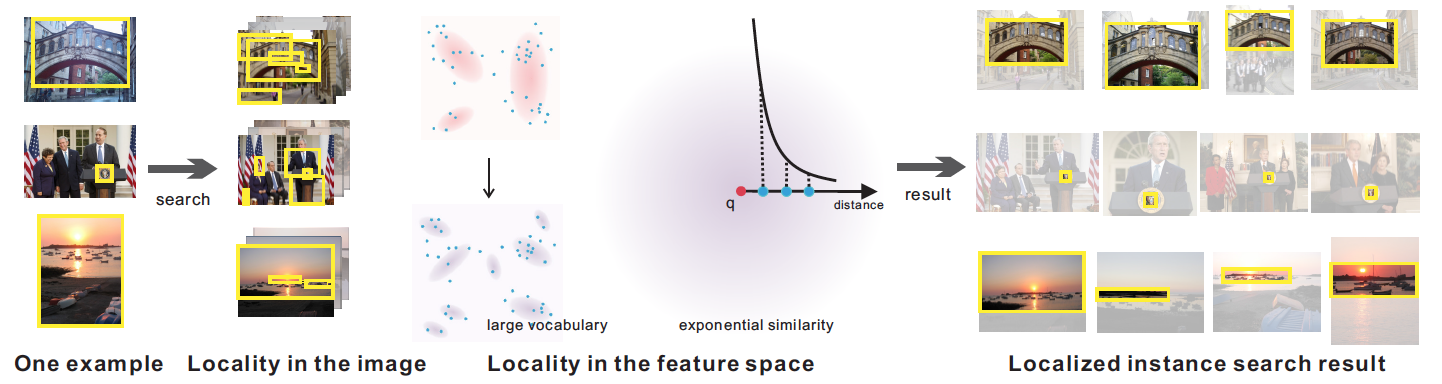
@InProceedings{TaoCVPR2014,
author = "Tao, R. and Gavves, E. and Snoek, C. G. M. and Smeulders, A. W. M.",
title = "Locality in Generic Instance Search from One Example",
booktitle = "IEEE Conference on Computer Vision and Pattern Recognition",
year = "2014",
url = "https://ivi.fnwi.uva.nl/isis/publications/2014/TaoCVPR2014",
pdf = "https://ivi.fnwi.uva.nl/isis/publications/2014/TaoCVPR2014/TaoCVPR2014.pdf",
has_image = 1
}