State of the art methods for image matching, content-based
retrieval and recognition use local features. Most of these still
exploit only the luminance information for detection. The color
saliency boosting algorithm has provided an efficient method to
exploit the saliency of color edges based on information theory.
However, during the design of this algorithm, some issues were
not addressed in depth: (1) The method has ignored the underlying
distribution of derivatives in natural images. (2) The dependence
of information content in color-boosted edges on its spatial
derivatives has not been quantitatively established. (3) To evaluate
luminance and color contributions to saliency of edges, a
parameter gradually balancing both contributions is required.
We introduce a novel algorithm, based on the principles
of independent component analysis, which models the first order
derivatives of color natural images by a generalized Gaussian
distribution. Furthermore, using this probability model we
show that for images with a Laplacian distribution, which is a
particular case of generalized Gaussian distribution, the magnitudes
of color-boosted edges reflect their corresponding information
content. In order to evaluate the impact of color edge
saliency in real world applications, we introduce an extension
of the Laplacian-of-Gaussian detector to color, and the performance
for image matching is evaluated. Our experiments show
that our approach provides more discriminative regions in comparison
with the original detector.
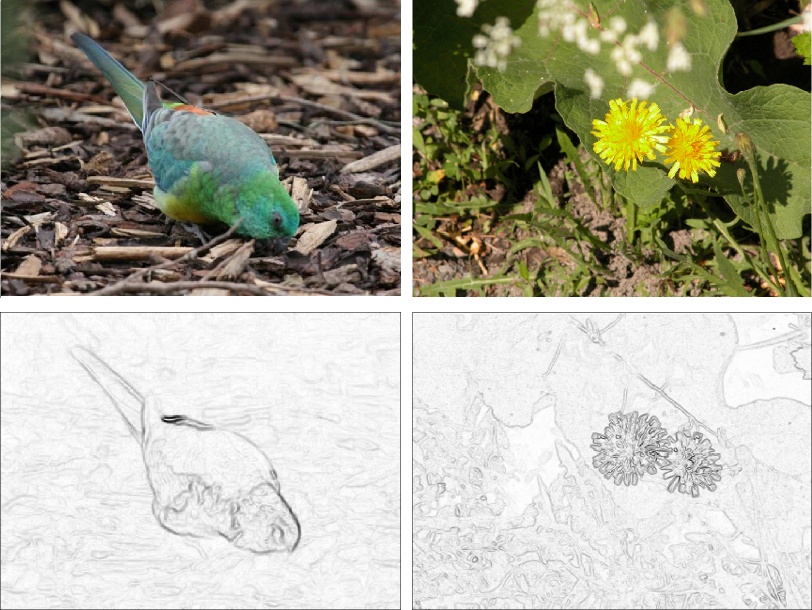
@InProceedings{RojasVigoECCGIV2010,
author = "Rojas Vigo, D. A. and van de Weijer, J. and Gevers, T.",
title = "Color Edge Saliency Boosting Using Natural Image Statistics",
booktitle = "European Conference on Color in Graphics, Imaging and Vision",
year = "2010",
url = "https://ivi.fnwi.uva.nl/isis/publications/2010/RojasVigoECCGIV2010",
pdf = "https://ivi.fnwi.uva.nl/isis/publications/2010/RojasVigoECCGIV2010/RojasVigoECCGIV2010.pdf",
has_image = 1
}