In this paper we aim for zero-shot classification, that is visual recognition of an unseen class by using knowledge transfer from known classes. Our main contribution is COSTA, which exploits co-occurrences of visual concepts in images for knowledge transfer. These inter-dependencies arise naturally between concepts, and are easy to obtain from existing annotations or web-search hit counts. We estimate a classifier for a new label, as a weighted combination of related classes, using the co-occurrences to define the weight. We propose various metrics to leverage these co-occurrences, and a regression model for learning a weight for each related class. We also show that our zero-shot classifiers can serve as priors for few-shot learning. Experiments on three multi-labeled datasets reveal that our proposed zero-shot methods, are approaching and occasionally outperforming fully supervised SVMs. We conclude that co-occurrence statistics suffice for zero-shot classification.
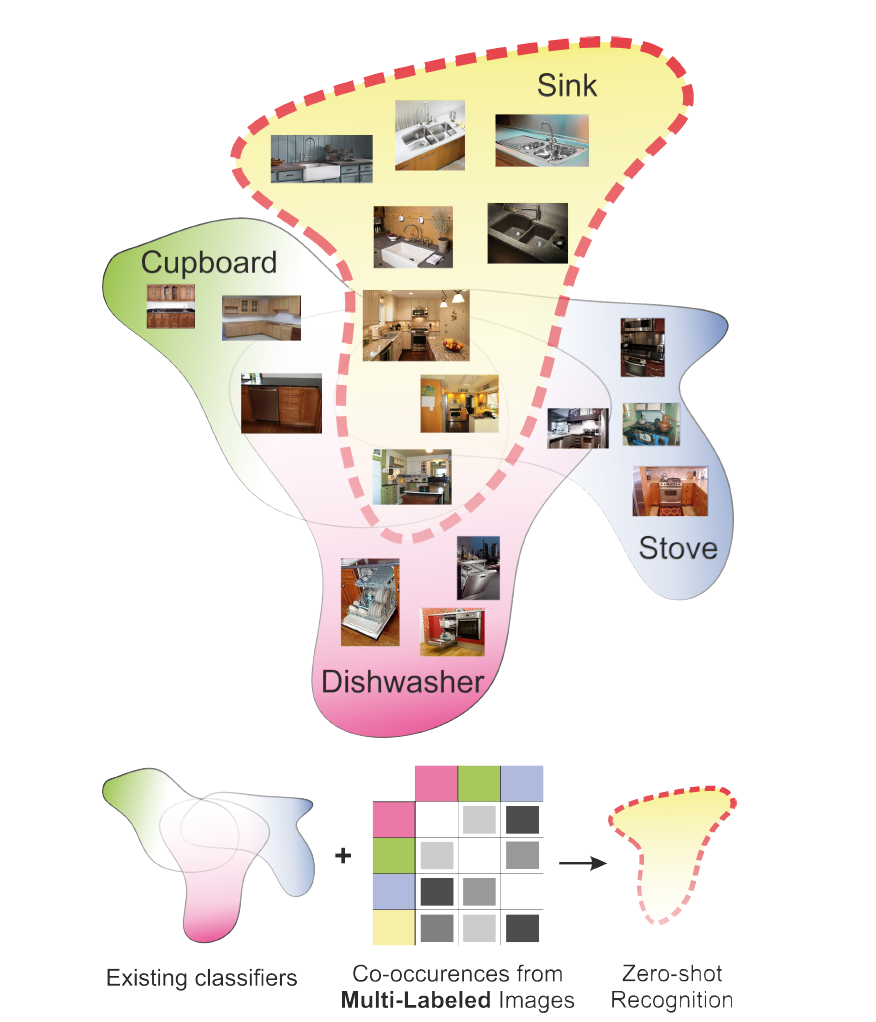
@InProceedings{MensinkCVPR2014,
author = "Mensink, T. E. J. and Gavves, E. and Snoek, C. G. M.",
title = "COSTA: Co-Occurrence Statistics for Zero-Shot Classification",
booktitle = "IEEE Conference on Computer Vision and Pattern Recognition",
year = "2014",
url = "https://ivi.fnwi.uva.nl/isis/publications/2014/MensinkCVPR2014",
pdf = "https://ivi.fnwi.uva.nl/isis/publications/2014/MensinkCVPR2014/MensinkCVPR2014.pdf",
has_image = 1
}