To learn classifiers for many visual categories, obtaining labeled training examples in an efficient way is crucial. Since a classifier tends to misclassify negative examples which are visually similar to positive examples, inclusion of such informative negatives should be stressed in the learning process. However, they are unlikely to be hit by random sampling, the de facto standard in literature. In this paper, we go beyond random sampling by introducing a novel social negative bootstrapping approach. Given a visual category and a few positive examples, the proposed approach adaptively and iteratively harvests informative negative examples from a large amount of social-tagged images. To label negative examples without human interaction, we design an effective virtual labeling procedure based on simple tag reasoning. Virtual labeling, in combination with adaptive sampling, enables us to select the most misclassified negatives as the informative samples. Learning from the positive set and a series of informative negative sets results in visual classifiers with higher accuracy.
Experiments on two present-day image benchmarks employing 650K virtually labeled negative examples show the viability of the proposed approach. On a popular visual categorization benchmark our precision at 20 increases by 34%, compared to baselines trained on randomly sampled negatives. The robustness of the proposed approach is verified by a cross-dataset experiment. The results clearly show the advantage of our approach: more accurate visual categorization without the need of manually labeling any negatives.
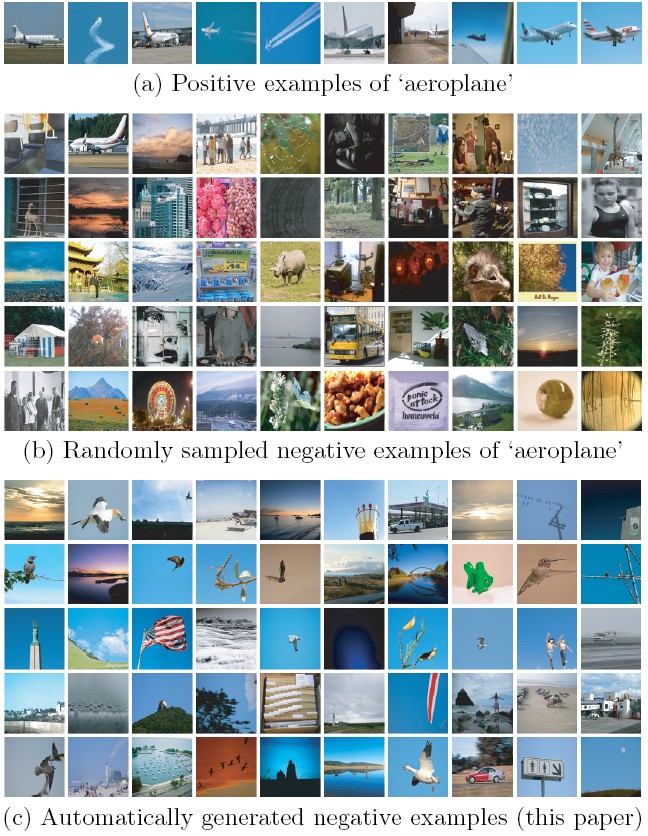
@InProceedings{LiICMR2011,
author = "Li, X. and Snoek, C. G. M. and Worring, M. and Smeulders, A. W. M.",
title = "Social Negative Bootstrapping for Visual Categorization",
booktitle = "ACM International Conference on Multimedia Retrieval",
year = "2011",
url = "https://ivi.fnwi.uva.nl/isis/publications/2011/LiICMR2011",
pdf = "https://ivi.fnwi.uva.nl/isis/publications/2011/LiICMR2011/LiICMR2011.pdf",
has_image = 1
}