Annotating the increasing amounts of user-contributed images in a personalized manner is in great demand. However, this demand is largely ignored by the mainstream of automated image annotation research. In this paper we aim for personalizing automated image annotation by jointly exploiting personalized tag statistics and content-based image annotation. We propose a cross-entropy based learning algorithm which personalizes a generic annotation model by learning from a user's multimedia tagging history. Using cross-entropy-minimization based Monte Carlo sampling, the proposed algorithm optimizes the personalization process in terms of a performance measurement which can be flexibly chosen. Automatic image annotation experiments with 5,315 realistic users in the social web show that the proposed method compares favorably to a generic image annotation method and a method using personalized tag statistics only. For 4,442 users the performance improves, where for 1,088 users the absolute performance gain is at least 0.05 in terms of average precision. The results show the value of the proposed method.
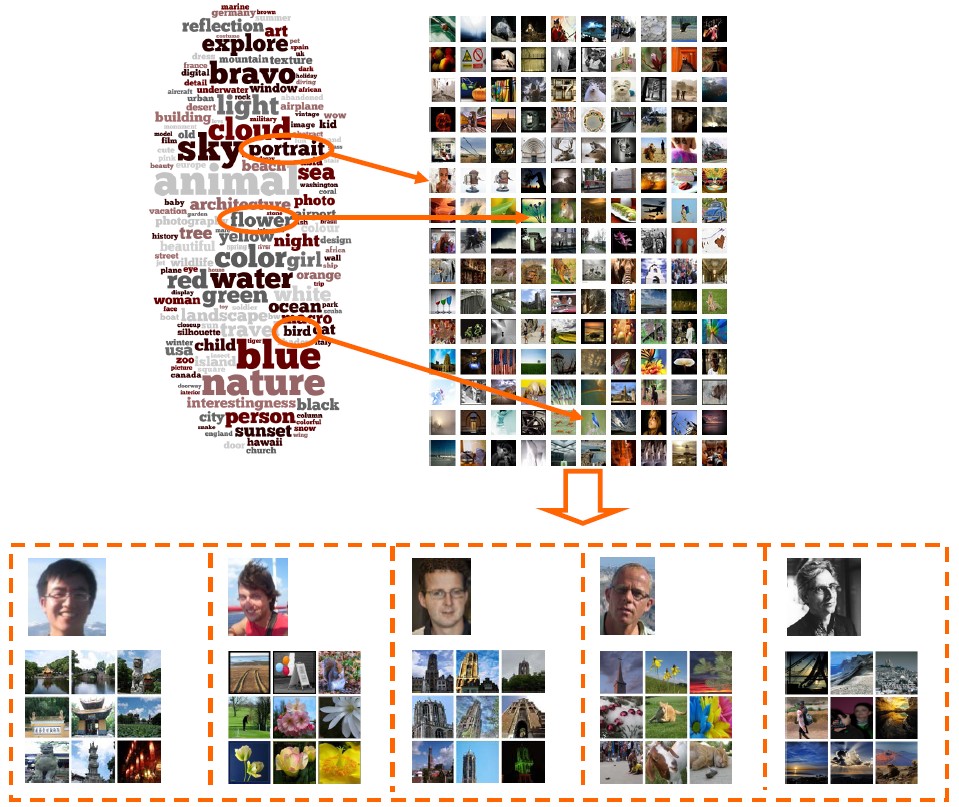
@InProceedings{LiICM2011,
author = "Li, X. and Gavves, E. and Snoek, C. G. M. and Worring, M.
and Smeulders, A. W. M.",
title = "Personalizing Automated Image Annotation Using Cross-Entropy",
booktitle = "ACM International Conference on Multimedia",
pages = "233--242",
year = "2011",
url = "https://ivi.fnwi.uva.nl/isis/publications/2011/LiICM2011",
pdf = "https://ivi.fnwi.uva.nl/isis/publications/2011/LiICM2011/LiICM2011.pdf",
has_image = 1
}