Web videos available in sharing sites like YouTube, are becoming an alternative to manually annotated training data, which are necessary for creating video classifiers. However, when looking into web videos, we observe they contain several irrelevant frames that may randomly appear in any video, i.e., blank and over exposed frames. We call these irrelevant frames stop-frames and propose a simple algorithm to identify and exclude them during classifier training. Stop-frames might appear in any video, so it is hard to recognize their category. Therefore we identify stop-frames as those frames, which are commonly misclassified by any concept classifier. Our experiments demonstrates that using our algorithm improves classification accuracy by 60% and 24% in terms of mean average precision for an event and concept detection benchmark.
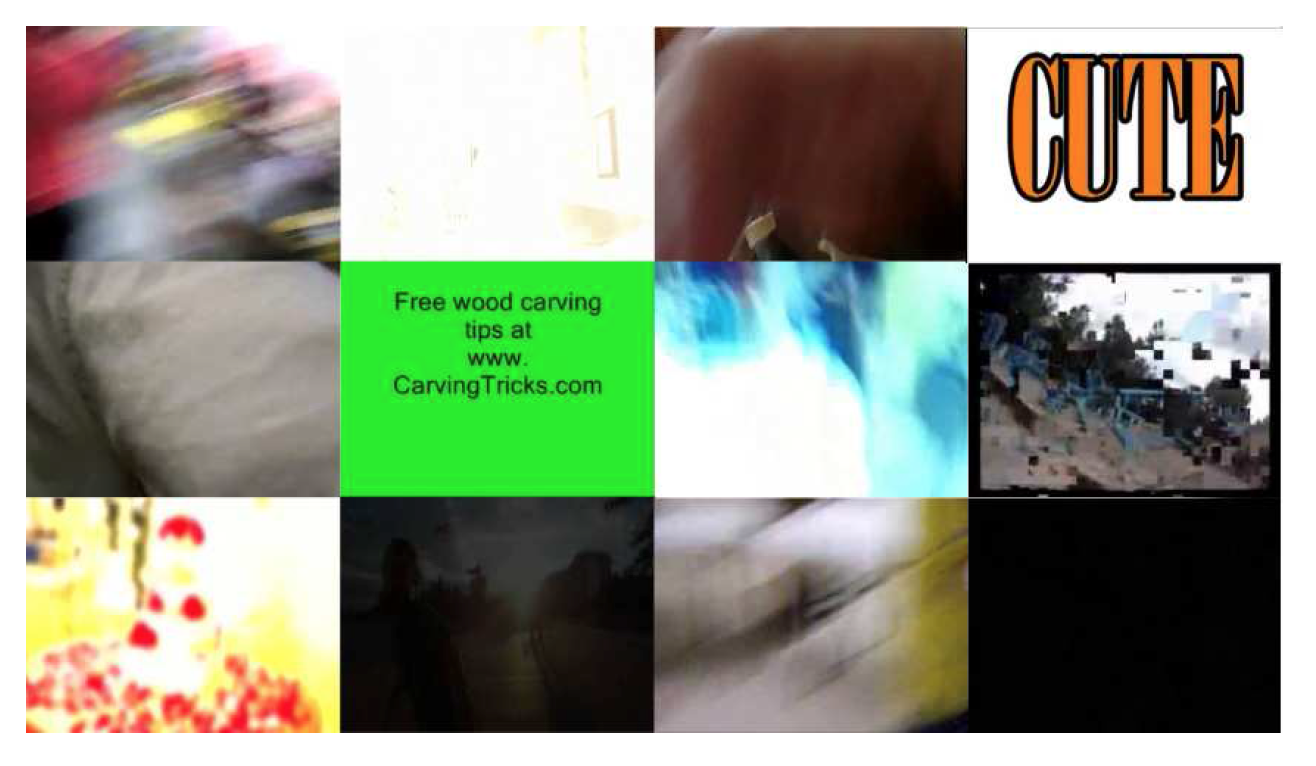
@InProceedings{HabibianICMR2014a,
author = "Habibian, A. and Snoek, C. G. M.",
title = "Stop-Frame Removal Improves Web Video Classification",
booktitle = "ACM International Conference on Multimedia Retrieval",
year = "2014",
url = "https://ivi.fnwi.uva.nl/isis/publications/2014/HabibianICMR2014a",
pdf = "https://ivi.fnwi.uva.nl/isis/publications/2014/HabibianICMR2014a/HabibianICMR2014a.pdf",
has_image = 1
}